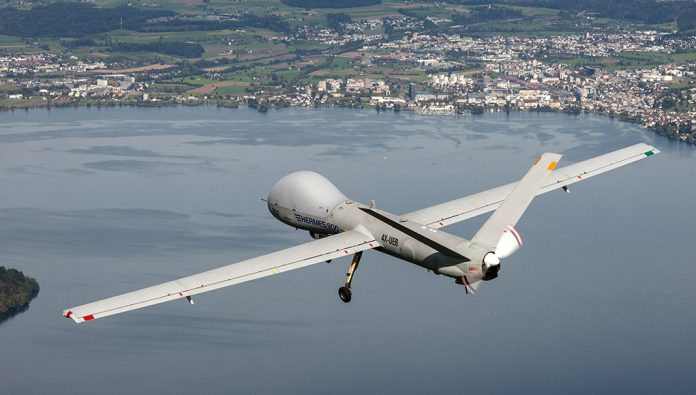
This post continues our discussion on Future Drones
Significant changes are required to make such autonomy a reality.
Trusted navigation
Inherent to their unmanned operation UAVs are dependent on external input for navigation and positioning. Global Navigation Satellite System (GNSS) is the most common, but not the only navigational aid. Unfortunately, such services are vulnerable to interference and exploitation, rendering military operation useless, if UAVs are not adequately protected. Use of other navigation aids, such as navigational beacons and odometry (distance measuring) is sometimes used, with limited success. Inertial measurement, Image-based navigation, even star tracking could also be used to augment existing navigation aids, in situations the GNSS data is not trustable.
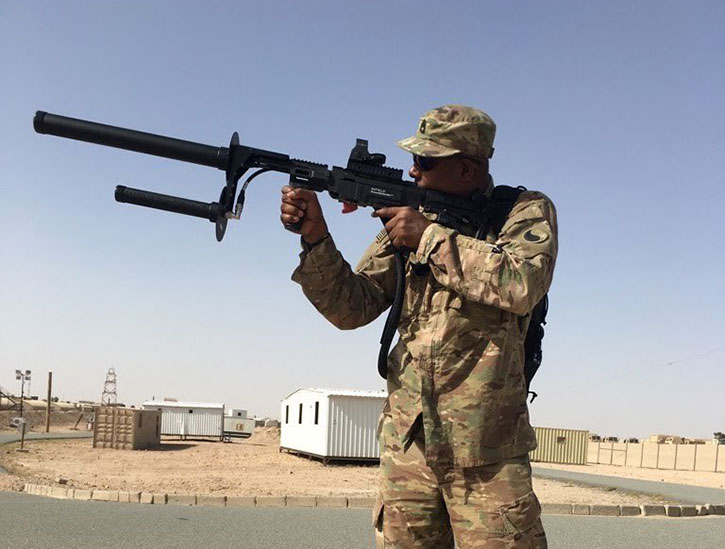
Communications and Security
Bound to operate under human control, unmanned platforms are dependent on maintaining a continuous link via terrestrial, airborne or space-based communications. The more sensors operate on board, the more bandwidth is needed to transfer the data they collect. High-capacity wireless communications links are relatively easy to jam because of their limited margins. High capacity links are often limited to maintain a line of sight with other nodes – further limiting the platform’s operation. Datalinks must also be highly immune. In the past, UAV communications channels used open analog links, but these are mostly obsolete today, as new digital links entered the field. However, datalink channels are still vulnerable to enemy attacks – jamming, interference, deception or exploitation via cyber-attacks. Overall, minimizing the platform’s dependency on external communications would render drones less vulnerable to jamming and more immune to exploitation, deception and ‘hijacking’ by cyber-attack.
Without a human presence on board, standard communications will suffice in operations in denied airspace, where communications and navigation are under electronic attack. Enhanced communications will increase survivability in a communications-denied area while minimizing aircraft detection. Advanced communications methods could include very short bursts to transmissions transferring information to and from the aircraft, enabling drones to receive and transmit signals over a very wide band, via a variety of communication mediums. When self-diagnostics detect a malfunction on board, the aircraft should be able to determine the most appropriate course of action, to continue the mission or return to safety.
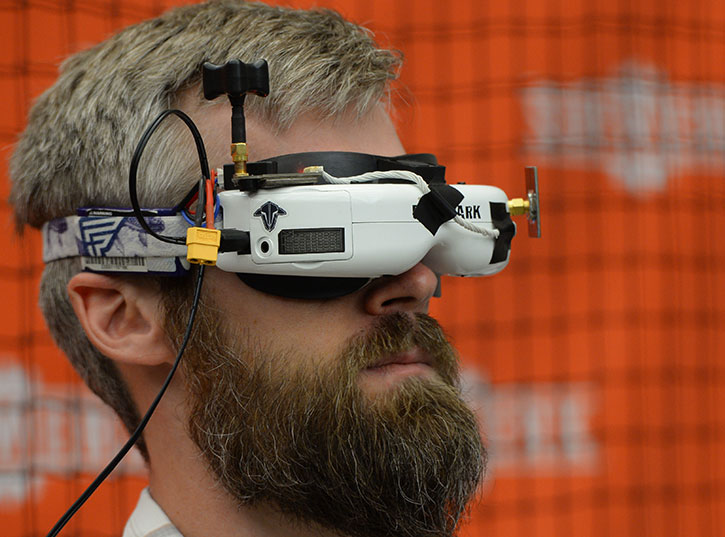
Command and Control
Today’s drones depend on the human operation, at least, for part or all their missions.Some platforms support autonomous operation for most of their missions, where the human pilot or operator’s functions are mostly supervisory. Others rely mostly on remote operations by trained pilots, using automated flight modes only as a backup. Both methods of operation consider the human pilot the highest authority on the mission, even though they may not have the best situational awareness and fast response needed to deal with emergencies. Introduction of fail-safe systems and situational awareness devices, such as sense and avoid (SAA) sensors, along with deep learning and artificial intelligence processors on board, will enable ‘smart autopilots’ to deal with emergencies faster and more effectively than the human, resulting in safer, more reliable operation.
The US Air Force evaluates such future capabilities under the Cooperative Automated Multi-Aircraft Operation (CAMARO) concept that defines evolving technologies – including multi-aircraft control, loyal wingman and drone swarms. All need autonomy, cooperation, and enhanced communications.
These ‘thinking machines’ could work with military drones but are yet to be adopted in the commercial world, since there are no procedures to certify such advanced routines to civil flight regulations. Therefore, innovators had to invest limited funds to implement specific functions that contributed to the mission efficiency and reduced losses but were not able to transform the market.
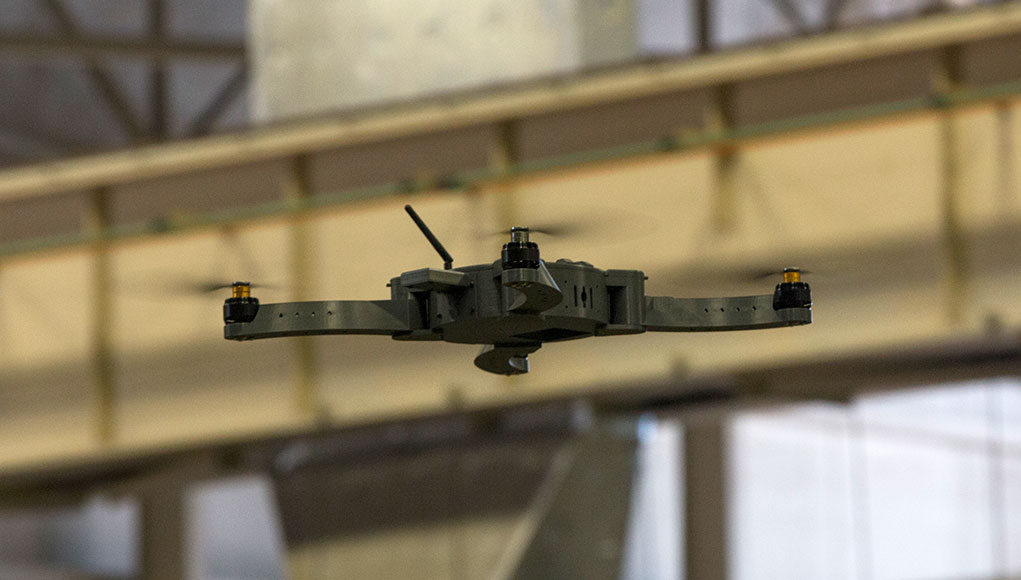
Assured, Affordable Autonomy
UAVs have limitations on the size, weight and power consumption of the payload and limited range and endurance. These limitations cannot be overlooked and are particularly relevant when considering deep learning algorithms to be run onboard.
Deep learning is recently showing outstanding results for solving a wide variety of robotic tasks in the areas of perception, planning, localization, and control. Its excellent capabilities for learning representations from the complex data acquired in real environments makes it extremely suitable for many kinds of autonomous robotic applications. In parallel, Unmanned Aerial Vehicles (UAVs) are currently being extensively applied for several types of civilian tasks, in applications going from security, surveillance and disaster rescue to parcel delivery or warehouse management. In this paper, a thorough review has been performed on recent reported uses and applications of deep learning for UAVs, including the most relevant developments as well as their performances and limitations. In addition, a detailed explanation of the main deep learning techniques is provided. We conclude with a description of the main challenges for the application of deep learning for UAV-based solutions.
Despite their advanced capabilities, the deployment of autonomous systems in safety-critical military applications remains challenging and controversial. ”To become truly effective, robots must be trustable, and this still presents a major challenge for humans.” Sandeep Neema, Assured Autonomy program manager at DARPA explains. DARPA launched the Assured Autonomy research program in 2016 to establish trustworthiness at the design stage of Cyber-Physical Systems (CPS).
The program explores new concepts and tools to enable Learning Enabled CPS (LEC) to meet ’functional measures of assurance’ and safety goals that will make such systems more trustable. LEC is defined as a component, whose behavior is driven by “background knowledge” acquired and updated through a “learning process,” while operating in a dynamic and unstructured environment. According to Neema, testing autonomous systems that evolve through their life is becoming a major goal for the program.
“Historically, assurance has been approached through design processes following rigorous safety standards in development, and demonstrated compliance through system testing,” Neema explains, these standards were developed primarily for predictable processes involving human-in-the-loop systems, and don’t extend to self-learning autonomous systems. The program will prioritize solving problems in the militarily relevant autonomous vehicle space, but, eventually will become available for use in commercial as well.
More in the ‘Future Autonomous Drones‘ review:
- Step I: Minimizing Dependence on Human Skills
- Step II: Mission Systems’ Automation
- Step III: Higher Autonomy Becoming Affordable
- Step IV: Teams, Squadrons, and Swarms of Bots
More in the ‘Future Drones’ series: